Table Of Content
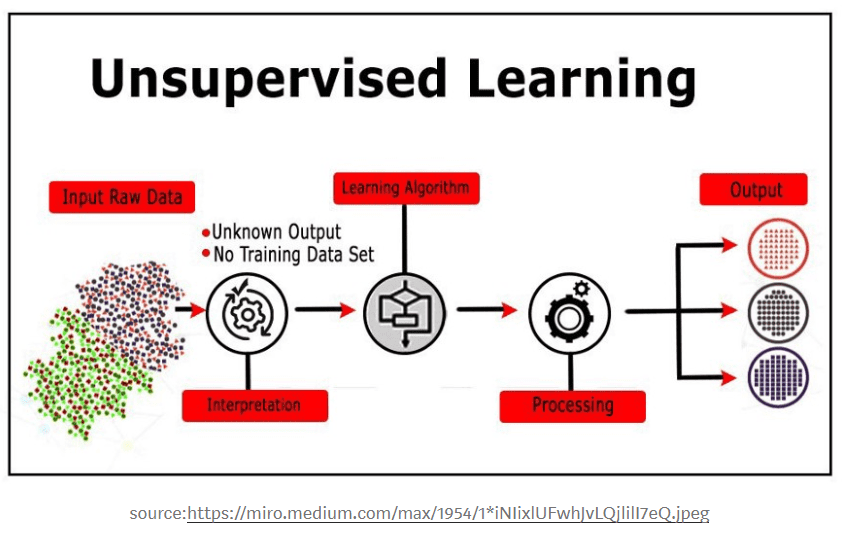
It would help if you discussed with the interviewer alongside these points. Another important thing is to analyze what kind of data is available to you and argue if there is enough versatility. You should be aware of the implications of the imbalanced dataset in ML and address it if need be.
A short tutorial on different normalization techniques used in Deep Neural Networks.
You’ll walk step-by-step through solving these problems, focusing in particular on how to design machine learning systems rather than just answering trivia-style questions. If you have a machine learning or system design interview coming up, you’ll find the course tremendously valuable. Top tech companies ask system design interview questions to see if you can efficiently solve real-world problems. Today we’ll discuss how you can ace machine learning interviews using system design concepts. I’ve also consulted several startups on their machine learning hiring pipelines. Hiring for machine learning roles turned out to be pretty difficult when you don’t already have a strong in-house machine learning team and process to help you evaluate candidates.
Training data collection strategies
I really found the quizzes very helpful for testing my ML understanding. Also, the resources shared helped me a lot for revising concepts for my interview preparation. This course will definitely help engineers crack Machine Learning Engineering and Data Science interviews. Interviewers will generally ask you to design a machine learning system for a particular task. The first thing you need to do is ask questions to narrow down the scope of the problem and ensure your system’s requirements.
Resources
Don’t forget that you’re not only trying to please the interviewer, but the panel of people who will be reviewing your performance later. Don’t give up any opportunities to show your experience and skills. Remember, unlike other interview rounds, you’re driving this interview, and you want to show both technical knowledge and leadership skills. After you've finished this book, you might want to checkout the 30 open-ended questions to test your ability to put together what you know to solve practical challenges.
In each later stage, you continue to increase the complexity (i.e. more optimized model in prediction) and execution time. The model needs to run on a reduced number of documents as the stages progress (e.g. your first stage could use a linear model and the final stage can use a deep neural network). In this article, we looked at an organized way of answering an ML System Design question. There is no one correct answer, and the purpose of this interview is to analyze the candidate’s thought process for designing an end-to-end system. Having said that, an in-depth understanding of various ML topics is necessary to succeed in this interview. Before you even begin working on the problem, you have to make sure you have enough information.
Books
These metrics will differ depending on the problem your system is trying to solve. Make sure you bring up how you would launch the system and actually evaluate whether it’s achieving its business objectives. This is almost always via A/B testing, which has lots of its own nuances.
Infrastructure Components
News Feed ranking, powered by machine learning - Facebook Engineering
News Feed ranking, powered by machine learning.
Posted: Tue, 26 Jan 2021 08:00:00 GMT [source]
These papers show there’s a wide range of ways to create state of the art recommenders, it’s definitely not a cookie cutter problem. Pay attention to the setups, how they do offline and online evaluation. Incorporate feedback on areas to focus on and see if they bring up red flags or criteria you’ve missed. This is also a spot to make sure you’ve fully understood the problem setup, before you get too deep in a solution.
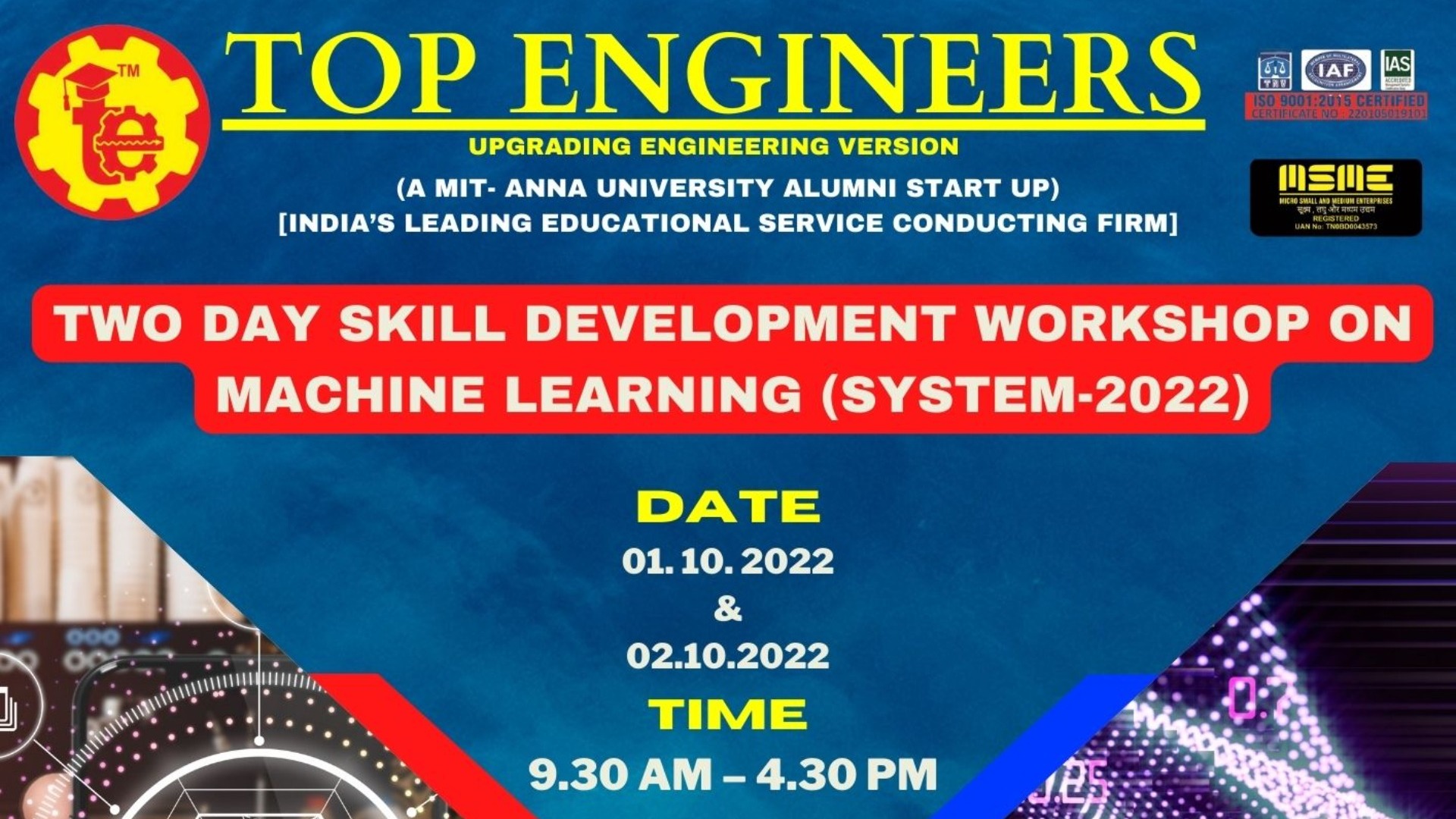
In a machine learning interview, you’ll be asked open-ended questions to test your ability to solve an ML system design problems, similar to system design interviews. This part also explains the interviewers’ mindset and what kind of signals they look for. One of the trickiest interview rounds for ML practitioners is ML systems design. If you’re applying to be a Data Scientist, ML Engineer or ML Manager at a big tech company, you’ll probably face an ML Systems design question.
ML Concepts
Talk about which metrics you’d measure and statistical tests you’d perform for an A/B test. You can go into some depth talking about ramping patterns and issues that arise with A/B testing. It’s not always a good idea to throw the kitchen sink at your model. Discuss some techniques for feature importance ranking and selection. Bear in mind this is fairly high level and abstract since you don’t have the data in front of you. You can also discuss regularization when you start to talk about models.
The quality and quantity of training data is a big factor in determining how far you can go in your machine learning optimization task. Data collection techniques primarily involve user interactions, human labelers, or specialized labelers. For the most part, I found that I wasn’t grilled too ‘deeply’ (pun) on deep learning. There are lots of ML positions that don’t touch deep learning. This is a pretty wide list of the topics that may come up during an interview.
How To Get A Machine Learning Job In 6 Months - Towards Data Science
How To Get A Machine Learning Job In 6 Months.
Posted: Mon, 08 Mar 2021 08:00:00 GMT [source]
I spoke to a lot of companies during my interview process including Pinterest, Spotify and Facebook. To be sure, this isn’t comprehensive so my experiences won’t apply everywhere! I’m not going to break my NDAs and say any of the exact questions I was asked, but I’ll give an overview. Compared to standard software engineering loops, there’s more variation between how each company evaluates candidates on ML skills. Some companies blended the questions with regular distributed systems designs while others focussed more on theoretical ML.
No comments:
Post a Comment